How Can Investors Use Machine Learning to Pick the Right Startups?
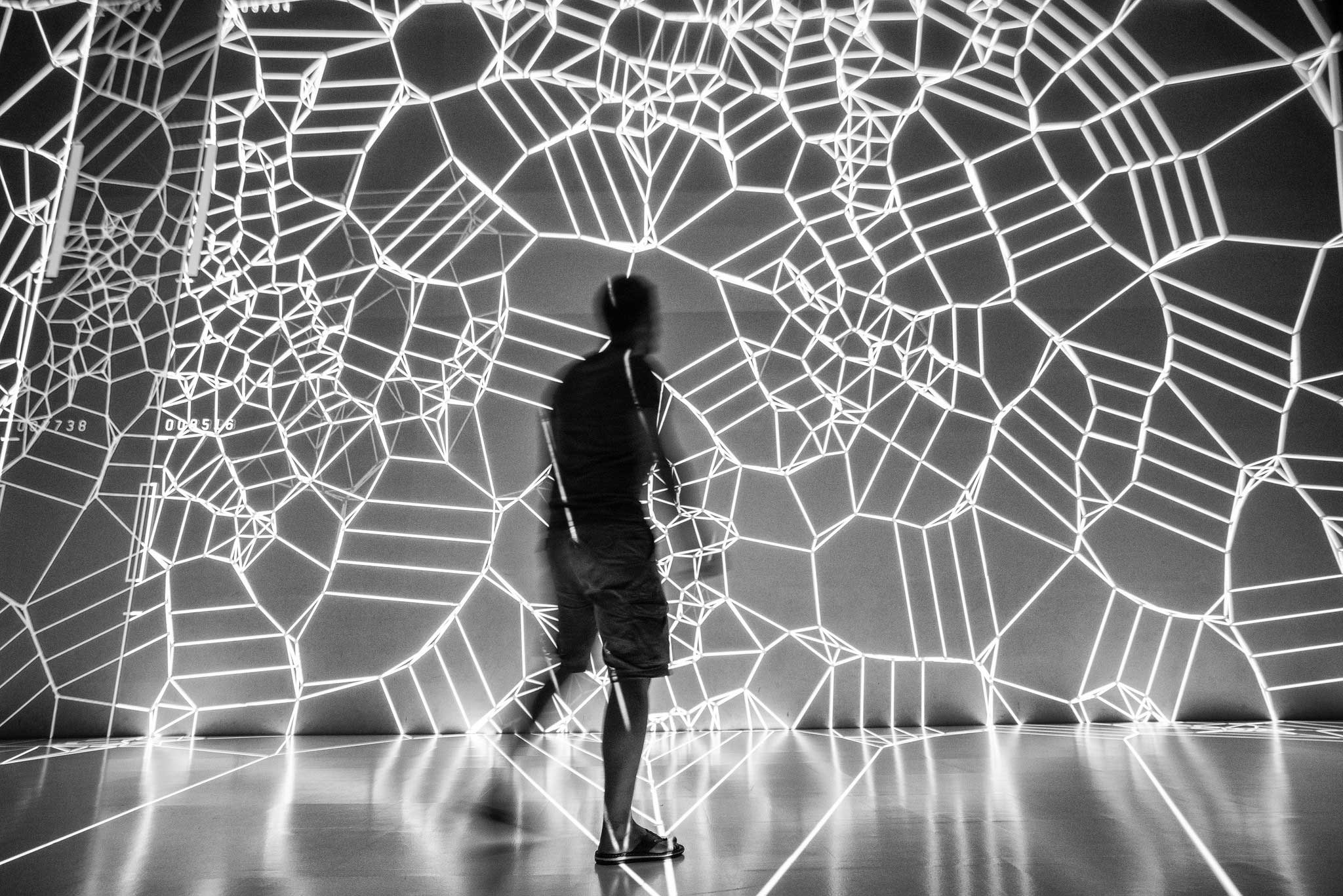
When considering a startup, especially an early-stage startup, investors want to conduct as much due diligence as possible. What little data they can gather is scattered all over different sources including Crunchbase, LinkedIn, Pitchbooks, company websites, etc. Consolidating this data takes a great amount of time and effort. Furthermore, the data sets can be incomplete or biased depending on the search queries — imagine overlooking a keyword. To make the due diligence process fairer and less cumbersome for investors, various platforms are using machine learning (ML) to pull together information about startups from all available resources to help investors assess companies and investment opportunities. But where machine learning really shines is in the interplay of data-driven insights that are qualified by human intuition and personal experience.
Take Hone Capital in Silicon Valley. Hone Capital partnered with AngelList to help them identify top deals by pulling information from several sources including Crunchbase, Mattermark, and PitchBook Data. Through this partnership, Hone Capital has been able to quickly ramp up sourcing deal flow to up to 20 deals per week early into its existence. When they compared metrics across the portfolio, the team saw its best performance from a combination of using recommendations from the humans on their team and the machine learning model.
Likewise at Catalyst Fund, we have taken advantage of a new ML platform called PreSeries, a joint venture between BigML and Telefónica Open Future, to evaluate very early-stage startups in emerging markets. PreSeries provides Catalyst Fund with a score for each company undergoing our investment selection process. The score represents the likelihood that the startup will go IPO, be acquired, hit a hard stop, or shut down. At Catalyst Fund, we have started using the score as an additional metric to complement our own due diligence process and to track the startups’ performance over time. PreSeries computes the score using the number of funding rounds, months to first funding, number of patents, months since last funding, whether a co-founder is the CEO and other variables. For example, the more funding rounds a startup has, the higher their score will be. The fewer number of months to first funding, the likelier the company will have a successful exit. Perhaps reflecting the sentiment of some Silicon Valley founders, PreSeries spews out a lower score the more MBAs there are on the startup team.
One of our portfolio companies — Destacame, an alternative credit scoring platform in Chile — currently holds a score of 52, dropping from its initial score of 67 in November 2016. At the time, Destacame had had two funding rounds, 660 days to first funding, three cofounders, and three MBA graduates. Since then, the number of days since last funding increased from 210 to 493, which might be driving the drop in the PreSeries score. However, in the interim period, Destacame has served over 500,000 customers, received over US$2M in funding, grew their team from 15 to 24 employees, and recently expanded to Mexico. These achievements are not reflected in the current PreSeries score and yet they are critical pieces of information for an investor. As such, the conventional factors that investors look at such as team members, traction, market opportunity, and innovation still need to play a critical role in the decision making process.
Siempre un lujo escuchar a @aficionado, un crack y CEO de @bigmlcom, que qu
iere poner un punto de realidad a los logros de la #AI en #RetinaLTD pic.twitter.com/knhOzEh3vm
— ⛓ José Luis Núñez ⛓ (@jota_ele_ene) November 28, 2017
Francisco Martin, CEO of BigML speaking at the RetinaLTD conference ,“Science fiction far exceeds the reality of artificial intelligence”
While ML has great potential to become a powerful and efficient decision making tool for investors, it’s still far from being perfect, or appropriate as a black box solution. What machine learning is helping investors to do very well is to check their biases revealed through more robust data, and increase operating efficiencies. Thus, valuable human time can be spent on the critical part of due diligence, where gut and judgement matter. Will machine learning ever be superintelligent enough to be the sole decision making tool for early-stage investors? Let us know what you think in the comments below.