Three common mistakes startups make when running lean experiments – and how to avoid them
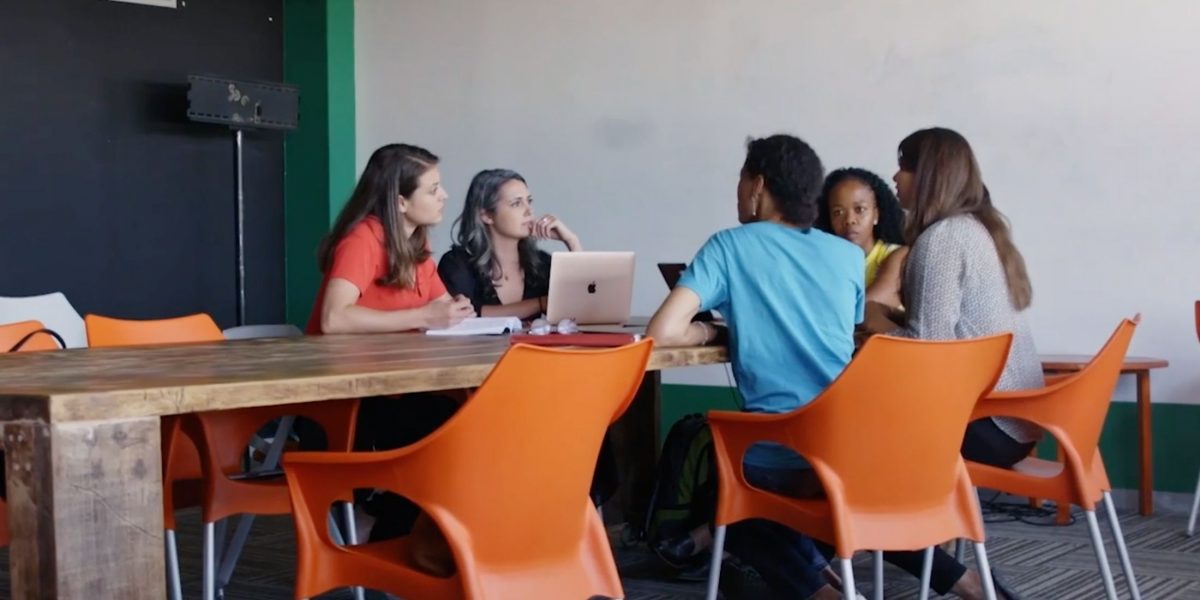
Originally published on NextBillion
Reaching product-market fit is essential for startup growth, but the journey can be chaotic, as founding teams are solving multiple problems at once. Startup teams need to create a product that is easy to use and can gain early traction, while identifying the right customer segments and power users, defining a marketing strategy, keeping costs low and conserving runway.
While planning and strategizing can generate insights into some of these issues, the most robust solutions come from experiments and iterations. At Catalyst Fund, we help our portfolio startups adopt a lean experimentation approach to accelerate the process of iterating their products. Explained simply, lean experimentation is a product development approach that helps startup teams swiftly turn ideas into products or services using a series of carefully designed and measurable experiments. This approach helps startups adopt a “build, measure, learn” approach, which can help them avoid wasting valuable time and effort building something that will not work. As Catalyst Fund’s Head of Portfolio Engagement Javier Linares says, “The most successful products we see are from those companies that recognize all they have are hypotheses (and not beliefs) and are running experiments to validate those hypotheses.”
We have shared the tools and templates we use for lean experimentation, as well as other aspects of venture building (like user research, marketing and more), in our improved product-market fit toolkit (available for free).
The toolkit can help startups understand what they need to do, but it’s equally important for them to avoid some common mistakes. Below, we’ll discuss three things startups should be conscious not to do when implementing lean experimentation.
1. CREATING UNMEASURABLE OR UNREALISTIC TARGETS
The essence of lean experimentation is to be aware of your hypotheses, clearly state them, outline potential tests and then define an objective measure of their success. When setting up an experiment, it’s important to define the outcomes you expect to see if the strategy is successful. In other words, it’s necessary to articulate what success looks like and how you will recognize it. To that end, here’s what a structure might look like, in general terms:
- We believe X.
- To verify X, we will …
- We will measure …
- We are right if …
Running an experiment without the foundation to track success is a recipe for failure. The key to successful experimentation is having access to timely, actionable data. Defining a core north-star metric for success is the first step teams can take to determine what their tracking efforts need to line up against.
Too often, teams implement a solution — often with a great deal of effort and planning — but have no way to evaluate the impact of that solution. Instead, founders rely on their instincts or anecdotal feedback to evaluate its efficacy. Other times, founders expect solutions to become silver bullets, and when their expectations aren’t met, they ignore the rest of the story the experiment is telling. However, an experiment that didn’t meet expectations is not a loss — it will often indicate the direction founders should take for the next experiment.
We’ve created a step-by-step guide on defining clear value propositions and hypotheses before running a lean experiment. We have also identified seven key charts that consolidate the critical startup metrics that every CEO should be asking their team to develop today, using off-the-shelf tools like Metabase or Redash. Such charts may provide founders with visibility into the impact of their experiments.
2. TESTING TOO MANY VARIABLES
Imagine you have a new product and you want to test two different marketing campaigns to see which is more effective. Within each of those campaigns, you may choose to test the copy for the ads, different media formats (such as images, videos, GIFs, etc.) or different calls-to-action. However, if you decide to change more than one variable within the same experiment, it will be difficult to determine which variable successfully contributed to the outcomes observed. This will make the experiment convoluted and will not give a clear indication of what actually worked or didn’t.
For teams looking to test multiple variables, we suggest running multiple A/B experiments, even with short timeframes or small samples, so that each winning strategy can be incorporated into subsequent experiments to determine the most successful combination. This is fundamental to smart, effective iteration and will ensure your startup makes progress toward product-market fit.
While we understand that mastering A/B experiments can take time, the more your team tests, the savvier they will get. Note that it is also important to have a defined control group in each experiment to compare against the treatment group. This helps you to effectively benchmark where you are and assess results.
For example, our portfolio company Cowryrise was looking to design a referral program that would delight their customers. They conducted an experiment to better understand what would nudge their customers to refer their friends to the startup, ensuring a control and single variable test in each iteration of the experiment. The design changes Cowrywise experimented with are outlined in a case study that describes how the startup collected important product and customer insights to better inform their referral strategy.
3. FAILING TO END AN EXPERIMENT
It is tempting to continue experiments “just a little while longer” in the hopes of getting better results. The problem is that when left unchecked, weeks can easily turn into months, unnecessarily risking precious time and resources. Instead, it may be useful to set a time limit at which point you will evaluate the outcomes of your experiment. It is better to string together many quick experiments, rather than allowing them to bleed together into an ongoing stream.
Perhaps even more significant is the need to dedicate time to synthesize learnings — i.e, to identify the key lessons you’ve learned from an experiment. The objective of an experiment is to not only identify changes you can make to improve your product but also to maximize learnings for future iterations. Companies tend to spend the least amount of time on synthesization, then rush to move on to the next experiment. However, an experiment that doesn’t generate lessons, whether positive or negative, isn’t a valuable experiment.
In addition to the product-market fit toolkit, our full stack of toolkits can help startup teams gain insights into their businesses and understand where to focus their efforts. For instance, our AI-readiness toolkit helps teams understand whether they’re ready to implement advanced technologies. Our risk diagnostic tool can enable founders to better map their top external and internal business risks and use our tailored strategies to best mitigate them. Given the impact of COVID-19, many early-stage companies have had to further adapt their product development strategies for a remote environment. In collaboration with GMC coLABS, we share case studies on six startups that have successfully leveraged product experimentation to continue their journey toward product-market fit remotely. These insights and toolkits can help startups implement lean experimentation while avoiding some of the pitfalls many founders face in getting their businesses off the ground. We hope you’ll explore our toolkits, and that they’ll be useful in your own work.