Could generative AI have helped in the recent floods in the Horn of Africa?
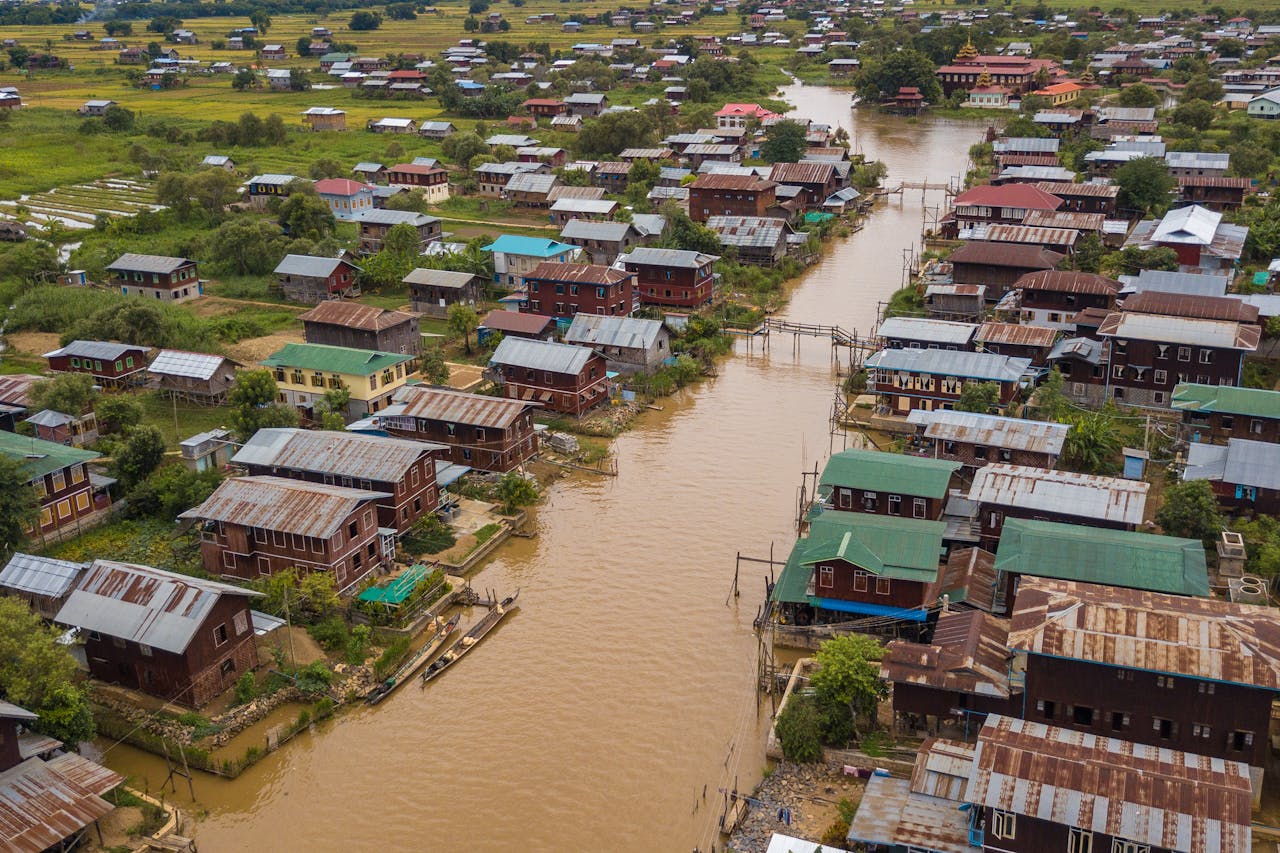
I was due to travel to our HQ in Kenya mid last year, so I have attentively followed the progression of predictions about El Niño causing floods in the region. Early warnings released in August evolved into devastating floods by mid-December, resulting in 170 lives lost and over 600,000 people displaced.
While following the news, a question arose: how could modern technology, specifically generative AI (GenAI), aid in these situations?
This article explores the role of GenAI in enhancing disaster response. It begins with a timeline of the recent floods in Kenya to show there is time to prepare communities better to face the disaster. It then looks at the availability of technology, such as accurate models to predict hazards and systems, processes, and knowledge to communicate with the local communities. Finally, it proposes an implementation strategy, pointing out existing opportunities and challenges and suggesting a way forward.
The timeline: from the announcement in August to live and economic losses in December
In August 2023, the Intergovernmental Authority on Development (IGAD) warned that El Niño would likely produce heavy rains and floods in the Horn of Africa, including Kenya. In October, the Kenyan Meteorological Department (KMD) issued a warning. On November 10th, the Kenya Red Cross activated the Early Action Protocol for riverine floods, which saved many lives and prevented more significant losses but could not avoid 170 people losing their lives and over 600,000 displaced due to this extreme weather event in Kenya by mid-December
The impact of these hazards goes beyond the specific situation in Kenya and the Horn of Africa at the end of 2023. Floods, storms, and heatwaves are responsible for most weather-related deaths (50%) and economic damages (79%), according to the World Meteorological Organization (WMO) data specific to Africa.
Weather models are improving, alert systems are disseminating information in the field, and fintech provides financial help to prepare for disasters. Is GenAI the secret ingredient to multiply the positive impact of these technologies?
These warnings were issued promptly, partly because floods, storms, and heatwaves are hazards that can be better forecasted and partially because models improve their precision and availability. Initiatives such as Graphcast are open-sourcing models that are more accurate in 99% of their predictions. Floods that started in October 2023 in the Horn of Africa highlighted how forecasting these events is possible with enough anticipation and great precision, and we expect both aspects will improve the existing hazard-specific models such as FloodHub. Can we employ generative AI to use this additional time to support communities to get better prepared?
The early action protocols already in place include disaster risk reduction measures such as disseminating early warning messages to at-risk communities. These messages reach numerous people but are unidirectional, non-interactive, and have limited personalization, as shown in the examples of SMSs below. These SMSs sent in April 2021 are part of the Early Warning for All activation plan in Tana River and amounted to 9,878,089 mobile messages. They were adapted to the county and provided in English or Kiswahili, but did not include any means for the recipient to get further assistance.
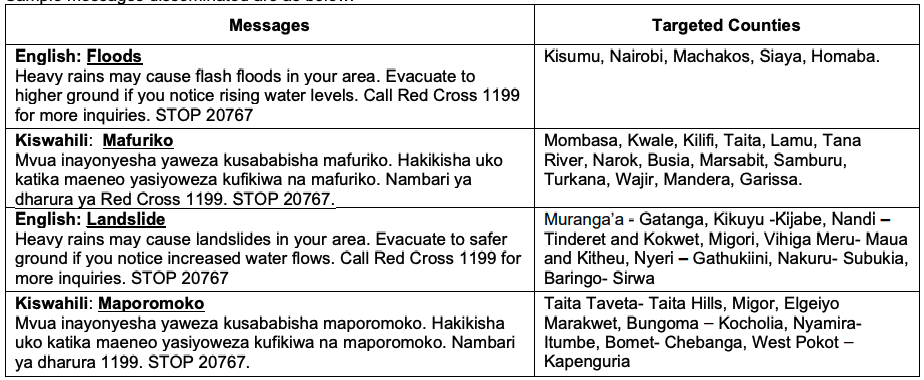
Can we increase the effectiveness of these messages, increasing the degree of personalization using GenAI and the learnings from past disasters? Can we include links to interactive services in those messages?
As members of the Universal Climate Resilience Co-lab in the CIFAR Alliance, we see how fintech solutions provide financial help directly to affected populations. Could GenAI help the recipients decide the best use of the funds received based on their specific situation?
We can imagine an interactive chatbot powered by GenAI that is able to help affected people prepare appropriate emergency kits based on the size of their family, helping them determine if evacuation routes are safe, or even providing a high-level suggestion on how to spend emergency funds. These chatbots need to be available via SMS or USSD as there is a significant prevalence of feature phones over smartphones in these communities over smartphones. Still, the smartphones available could provide richer information, such as maps, graphics, and icons (as pictured below). The mockup below, created by the CIFAR Alliance’s Universal Climate Resilience Co-Lab, shows an example of the type of guidance the systems could offer.
Despite the existing challenges, there are significant opportunities in the space
We have all heard stories about GenAI models hallucinating and making up information. We can imagine the nefarious impact this could have in an emergency. Still, we can overcome this limitation by fine-tuning the models with curated information and complementing the systems with additional guardrails such as filters for specific questions and escalation paths for critical topics. An opportunity to curate the existing content could involve volunteers trained by humanitarian organizations such as the International Federation of the Red Cross as part of their emergency plans. These humanitarians are the main task force that brings material help and information to affected communities. In 2021 alone, during the Kenya Floods Emergency Plan of Action, 320 volunteers were trained. Could we involve these volunteers as the first users of GenAI-enabled solutions? Could we implement Human-in-the-loop systems to help improve the systems and improve accuracy using their inputs?
Another challenge is language fragmentation; any proposed interactive solution needs to work in the languages spoken by the local populations, and can be designed to be implemented inclusively to address potential barriers such as lower literacy levels and visual or other physical impairments. Systems need to understand the questions and clearly express the answers so that the information is transparent to local populations. The existing models are not available with the same label of proficiency for text understanding and text generation in all the languages, and although there are models available for translating and understanding many written and spoken languages, like those included in initiatives like Seamless M4T from Meta, careful review of their accuracy in these domains needs to be studied. The good news is that local technical expertise is improving models for underrepresented languages. Initiatives such as the award-winning Masakhane Research Foundation and Lenapa AI are just two African examples. Another opportunity is English-speaking regions in Africa and India, which could benefit from an initial deployment of the solutions with smaller adaptation efforts to deploy the models.
A third challenge is how disasters can affect the infrastructure that holds these systems. Storms and floods can damage telecommunication infrastructure, potentially affecting the service, so systems should not only consider high availability strategies but also focus initially on alert stages where the infrastructure is unlikely to suffer damage. Newly released powerful open-source models can run on less powerful computers and even certain phones, which opens the door to having these disaster-specific models available without an internet connection in the nondistant future. We can think of specific devices available in certain community centers to support local leaders when the internet connection is unavailable.
Let us aim to increase the preparedness of the local population when the next disaster hits
Leveraging these solutions requires intense work and collaboration, but the impact could be enormous. In the recent events in the Horn of Africa, more than one million people were affected. Developers, policymakers, and humanitarian organizations need to join forces in refining these solutions:
- Developers can create the chatbots and ensure they work in the local languages, the specific communication channels, and the disaster preparation use case.
- Humanitarian organizations can help curate the content and learnings and involve trainers and volunteers to adapt it to the local communities.
- Policymakers must push for inclusion in the emergency plans and deploy solutions addressing the risks and limitations.
- Organizations responsible for deploying early warning systems are in the best place to coordinate the efforts, pursuing the integration with the most advanced meteorological data and creating the most impactful roadmap.
Acting together and harnessing the full potential of GenAI chatbots, we can strengthen our resilience to the inevitable challenges posed by climate disasters.
To inspire and encourage collaboration, we have made a GPT available with information specific to Kenya, such as the documentation for training the trainers, the emergency plan of action for 2023, and a copy of this post. If you have a GPT Plus subscription, we invite you to interact with the GPT and help us think about the problem and imagine how this technology can help in disaster scenarios like the ones we, unfortunately, faced recently. Reach out to me directly if you have any questions or feedback; we’d love to hear from you.