Catalyst Fund toolkit helps startups get AI ready
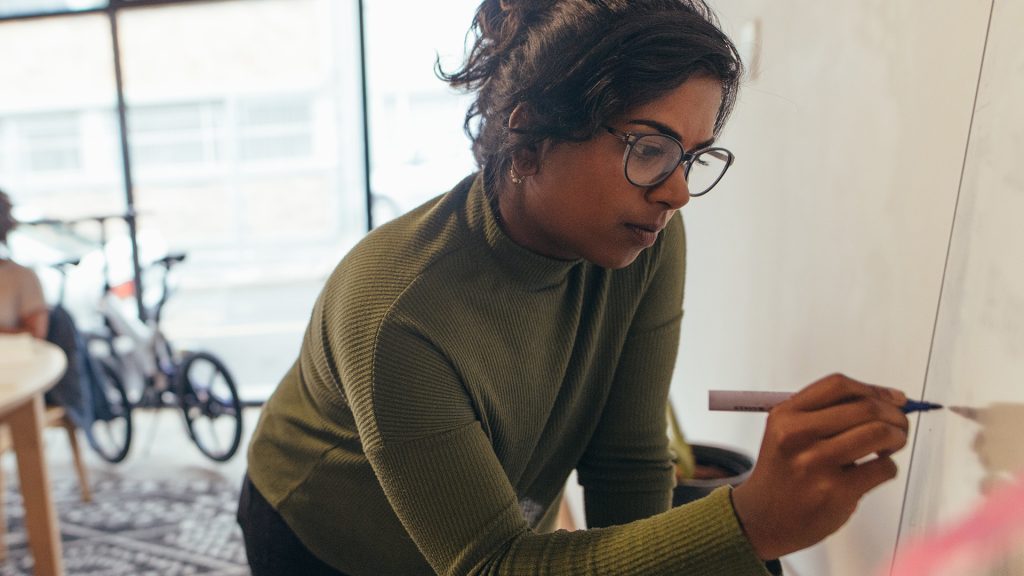
In India, we’ve seen a vast array of AI solutions deployed across all sorts of industries. Funding of AI startups is growing fast, from $113M in 2017 to over $760M in 2019, and trends suggest continued growth in the coming years. That said, when it comes to evaluation, adoption, and integration of AI solutions, leaders are often unsure where to begin, how much data is enough data and how to build on what they already have.
Last year, Catalyst Fund released an AI-readiness toolkit to help startups and other organizations assess data readiness and ease progress on their AI journey. We described the six steps to an intelligent data strategy in a blog last year, describing the data building blocks towards utilizing AI. The toolkit helps organizations assess their current status and chart an AI readiness path based on the pyramid below.
We recently shared our experience using the toolkit to assess different stages of the AI-readiness framework with startups at The Mumbai AI Summit. We have found that startups can use the toolkit to understand what level they’re currently at, and what they need to do next to implement AI.
This journey can be considered in three steps:
1.
Explore the data
The success of any AI solution lies in the type, amount and process by which data is collected, stored and analyzed. Understanding the state of this data is critical in setting the foundation for any AI-readiness journey.
As such, organizations need to start by taking stock of what they have, including an inventory of collected data points, the data’s relevance in decision-making processes, the suitability of their formats, where they’re stored, who owns them and their access management from a user and legal perspective. This review should also identify missing data that will have to be collected based on whichever channel makes sense: interviews, surveys, instrumentation of digital applications or other techniques. Then, data needs to be moved to appropriate storage through APIs or other tools, validated and tidied, and finally, put through some rounds of exploratory data analysis to extract useful insights and understand different relationships among data points.
This initial assessment can serve as a foundation for eventually training the AI model, particularly to generate initial insights. These insights could be related to understanding the customer served, e.g., learning about customer segments, or potential trends and patterns in their activities.
It is also very important at this stage to check for biases so as to avoid incorporating them into the AI model. Biases, for example, may come in through data sources that systematically over-represent or exclude certain populations due to unbalanced sampling. As data grows, these trends can be harder to identify and repair, and underserved groups may ultimately be excluded from the model’s decision-making process.
When payAgri joined Catalyst Fund, they were connecting farmers with various retailers and input-providers to ensure fair pricing and access. They had a strong network of agri-industry experts and practitioners, a compelling value proposition, key stakeholder buy-in and in-house sector expertise.
At that time, they had an exciting vision to develop an AI-based recommendation engine that would use data from IoT sensors to determine if farm crops needed fertilizer, water or other inputs. The smart system would be able to alert farmers when their crops needed attention, and therefore enhance farm health. Since payAgri was just starting out, they just had an initial sketch of sample data schemas that they planned to collect, monitor, and feed into the AI model.
Given their AI goals, Catalyst Fund helped to conduct a readiness assessment, which prioritized building out their data infrastructure to scale and structure the data to feed this algorithm. We refined their data sets and schemas, added checks for data validation to ensure clean data were gathered, reviewed the app and UI to streamline the data gathering channel and developed feedback mechanisms for farmers to feed inputs back into the system allowing for easier data analysis and learning. With these improvements, this data foundation could serve as the basis for the insights engine.
Based on this exercise and in part on the next several priorities in the readiness assessment, payAgri transformed their data model to focus on connecting farmers with different buyers in the agri-value chain. They have since doubled down on efforts to capture real-time farm-side data on the quantity and quality of farmer produce, as well as the buy-side data on prices at which farmers can sell. Their current AI vision is to enable price-predictions for farm produce so that farmers can make more informed sales decisions.
Key takeaway: The first step towards AI readiness is to develop the right data infrastructure and collection processes to create a robust data source to build models on – be patient and be thorough because “garbage in, garbage out”.
2.
Create the insights engine
This is where the fun begins. If the toolkit suggests your data is properly collected, organized, and stored, you can start to aggregate the data and begin learning through specific tests and hypotheses. These initial tests can help identify patterns in the data, assess predictive power and indicate the most effective direction to pursue toward a working ML model.
Organizations must keep in mind that — wherever possible — this insights engine should not be treated as a black box, and should make results and decisions transparent to users. For example, when it comes to building a credit-decisioning model, a binary approved/rejected result with no explanation can add confusion and a feeling of distrust among customers. Being transparent in the granular factors that inform a decision empowers customers to take informed actions toward their goals, to enjoy a much better credit experience, and as they understand more, leverage the product for greater impact and ultimately feel a higher level of trust and loyalty to the company.
BFA Global worked with Optimetriks to move from data collection towards insights. Optimetriks is a micro-merchants-oriented FMCG intelligence company focused on product availability in small shops mostly across East and West Africa. The company had the right stakeholder buy-in, a viable operational model and a robust agent network that collected information by taking pictures of products in stock at shops.
Together, these pictures constituted a rich, high-quality data source. Their agent network was responsible for sifting through the images and manually counting the types and numbers of products in the images to ultimately feed them into the system. Optimetriks would then use these counts to make predictions about future stocking needs.
Given this rich source of data, our intervention sought to leverage AI to optimize their model. We helped design an object detection algorithm to automate the process of identifying and counting unique products in images, thereby dramatically increasing efficiency. We tested the algorithm to assess how many labeled products were needed in the training set to maintain a certain level of accuracy, what level of obscurity in images would still yield accurate results and how non-products could be recognized without confusing the counting process. Each test had to be run multiple times with learning from the previous run incorporated in the new test cycle. Once the model was trained, it was embedded in a mobile app to be deployed using the smartphone’s camera.
We also supported the solution in the third step of deployment, determining how these insights could be used as a channel for alternative credit scoring in addition to the existing value-add of informing suppliers of product needs and providing discounted prices to the merchants.
Key takeaway: Once your data layer is ready, you can move to generating and testing initial hypotheses. Frequently testing hypotheses helps add context and experience to the ML model, so don’t stop testing!
3.
Consider organizational readiness
The culminating stage in the journey is preparing the organization to finally deploy the AI solution. Once the data is ready and the initial insights structure is conceptualized, organizations need to think about how to manage AI within their decision-making and operational processes. It will be important to monitor the algorithm using success metrics, compare these measurements against expected outcomes and keep a tab of unintended consequences (e.g., Are you cutting out some segments of the customer base? Is there a bug in one of your chatbot models? Is the model fair in terms of what you and your customers expect it to be?).
These questions and metrics should form the context within which the AI model can be deployed. Beyond managing the model, three other considerations at this final phase include preparing the technology (e.g., integration of the model into a mobile or web app), structuring the team (e.g., ensuring there are champions and specialists that own the roll-out and implementation processes) and managing operations (e.g., change, process, skills, and product management).
When Catalyst Fund started working with Smile Identity, an identity verification platform that uses facial recognition to perform KYC checks and biometric authentication, they were already on the path towards using AI in their business. They had already completed an impressive proof of concept leveraging 3D facial recognition technologies and had demonstrated their solution was highly effective in US-based testing. Furthermore, they had a dedicated computer vision and machine learning team, and executive buy-in to build a localized solution.
At the time, they were looking to do user acceptance testing with prospective users in Kenya and Tanzania, new environments where their solution was yet untested. They needed to understand market technical constraints (common device and OS types, camera quality and mobile internet speed) as well as user expectations for human-computer interaction. At the outset, the key success metric was accuracy: how accurate were models trained on U.S. datasets in uniquely identifying African faces? Were their models trained in a way that was appropriate and sufficient to maintain accuracy given the new types of face and ID images coming into the system?
Given that this was a new market, Catalyst Fund support started with helping strengthen the insights’ engine in the new context. We established user trials and interviews, and helped make recommendations on how to optimize their model using deep learning technology. We brought in more data sets with low-lighting and different skin tones. Critically, these trials exposed user behaviors in Kenya that the model was not yet prepared to handle. For example, the Smile software had used smiling as a trigger for image capture, but some people in rural Kenya did not want to smile when taking a selfie. These learnings forced adaptations that helped improve the consistency of image capture and ultimately the accuracy and expected outcomes of the solution once it was deployed.
Key takeaway: It is critical to continuously monitor and evaluate the AI solution by comparing measured versus expected outcomes, and monitoring for unintended consequences to ensure a successful deployment.
From working with startups – and also in applying the same principles with incumbent financial institutions and financial authorities – we’ve learned that it’s important to frame AI readiness and deployment as a journey, one that is iterative instead of a one-shot solution. Even once a model is developed and working, when you update a product, add a feature, deploy a new technology or expand to new markets, it is important to revisit the readiness framework.
In this day and age, it is common to seek out solutions powered by AI. That said, not all AI is created equal. Stakeholders need to ensure models are built using best practices, ask the right questions to identify relevant use cases, list the success metrics of the predictive model and define what they expect as their business outcomes before embarking on the journey that we described.
We will continue to refine our toolkit in the meantime, and invite you to try it out and provide thoughts and feedback that we can incorporate into future releases.