When the best available human is an AI?
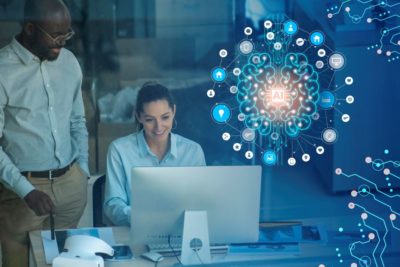
Developing country economies are characterized by shortages of skills that seem to be good for AI to fill: from tasks like writing and coding to, potentially, business advice and decision-making. But when do we decide that generative AI (GenAI) is actually better than the Best Available Human? The Best Available Human standard asks the question: would the best available AI in a particular moment, in a particular place, do a better job solving a problem than the best available human that is actually able to help in a particular situation?
Promise and opportunity
The Best Available Human in many emerging market contexts isn’t really up to the job; there’s a yawning mismatch between employees’ skills and what’s really needed. As this ILO report from 2019 indicated, “skills mismatch can negatively affect labor market outcomes, workers’ productivity, competitiveness and economic growth.” Management practices are worse, leading to worse performance for firms. We end up with a situation where employees need more supervision and coaching, yet managers are weaker and less competent.
GenAI to the rescue! More so than in mature markets, where skilled managerial and technical talent is relatively more available, GenAI seems to offer the promise to step in and take care of not just rote and routine tasks but tasks requiring technical skills, writing ability, understanding of complex subjects, and business acumen.
The line where GenAI becomes more useful than the Best Available Human is drawn earlier in emerging markets because the Best Available Human doesn’t have as much training and experience. To take accountants as an example, since maintaining accounts is key to the growth of any business, there were 25,589 registered accountants in Kenya in 2021, according to the National Treasury. Meanwhile there are 144,000 registered business entities and 1.5 MILLION formally registered micro and small businesses and over 5 million informal businesses. Not everyone needs a CPA but you can see that finding a qualified bookkeeper is probably not easy. Even Bill Gates thinks GenAI can help cobble over shortages of skilled workers like doctors and teachers.
Recent research seems to indicate that GenAI can be most helpful to mediocre and low-skill employees; if this is borne out in the real world, then GenAI could be a force that helps developing countries catch up in the productivity marathon. Whereas before it was prohibitive to find the capital, time and expertise to put in place automation, now GenAI’s near infinite capacity to learn, adapt and mimic should be able to augment low-skilled workers with much less drain on management oversight.
For instance, a startup could employ a GenAI to speed up coding a new product or a government could use GenAI to improve how they deal with customer service complaints. A small company could use AI services out of the box to automate document processing tasks as part of their accounting and consult an AIBot when it has business questions.
These are just examples that are already underway. And no doubt managers, leaders and entrepreneurs will find other uses that we don’t yet know about.
So that’s all great, and GenAI is going to solve all our human resources shortages, right? Not so fast: we’ve seen adoption so far is limited (except in the exam cheating field, but that goes unmourned) and there are good reasons for that.
- It’s actually not that easy to implement a GenAI application. Devising, testing, training and maintaining a GenAI application requires both a structured data set and also a kind of structured thinking that probably comes easier to engineers than to small-business owners with high school education. The investment may pay off in the long term, but mostly we’ve been promised easy, instant and cheap results.
- How do you know it’s actually better than the best available human? At BFA Global, we’ve tested GenAI to help us assess HR applications and startup pitch decks. GenAI recommends different candidates than our team does, but we can’t say they are better. Or worse, for that matter. That’s left us with a question about our own assessments – is human “gut feel” playing a bigger role than it should? Or conversely is it actually the most important thing and will never be outsourced to GenAI? At least, it has led us to think more about how the organize the task in a more structured way so we can better understand decision-making by humans and by GenAI.
- How do you know when to turn the GenAI back over to the human? Dave Holz’s recent study in Kenya indicates that while high performers improve their outcomes with GenAI, poor performers actually do worse than before, perhaps because they ask questions that GenAI just can’t answer and maybe provides misleading or unsuccessful recommendations. How do we know when we need to hand back the baton? And where expertise shortages are the reason why GenAI is used in the first place, is that moment earlier or later?
Recommendations for designing AI systems when the Best Available Human isn’t that available
People are going to adopt GenAI if they feel it has the potential to be helpful, so here are a few things that GenAI developers, promoters, and systems engineers should take into account:
- Local context awareness: Given that many AI systems are trained in Western settings, it’s crucial that they are designed to consider that local context and practices may be different; perhaps by prompting users to think and consult.
- Educational onramp: Given the general lack of education about AI, systems should incorporate educational guideposts to help users understand how to make the most of them, as well as their limitations.
- Affordability and accessibility: Increasingly, GenAI is going to be cheaper than the Best Available Human, further incentivizing adoption. But usability is still a challenge, so making systems as easy to use and as transparent as possible will be important.
- Handing back to the humans: GenAI should know when it’s no longer useful, whether because of the complexity of the question or the feedback from the user. It is possible to instruct the GenAI to be specially careful on certain topics, and this technology can be combined with other technologies ie: intent recognition that can prevent answering at all certain questions. In the end rather than confabulating increasingly unhelpful or even dangerous answers, it should give in and say, I don’t know, ask a Human.
In conclusion, GenAI is set to augment the humans we have, who often are not the humans we really need. At it’s best, we hope that GenAI will cobble over that gap and equalize human capabilities, allowing rapid catch-up for companies in emerging markets. It’s early days yet – probably in the short term, we’ll see that GenAI makes human capabilities better, but not as much as we need. And it will introduce new problems that we may not yet be set up to handle. It’s a journey of exploration and we expect that there will be quite a few twists and turns on the road.