Machine Learning in PAYGo: What You Need to Know Before You Jump In
Last summer, we published a post that described how ZOLA Electric uses Machine Learning (ML) to predict which of their customers are likely to stop paying. The post was informed by the work BFA’s FIBR Project has done with ZOLA, a leading PAYGo solar operator in Tanzania, Rwanda, Ghana, Nigeria and Ivory Coast. Since that time, many PAYGo operators in the solar, cooking and water sectors have contacted us asking for more information about how ML actually works in practice, and for guidance on how to get started.
In this post, we’ll describe three concrete steps to help your PAYGo enterprise prepare to implement ML. Before we get started, remember that ML is not a magical solution to business model problems. Rather, it is a statistical process that your team can use to learn from your experience. Simply put, ML allows you to put all the data you have collected to work by using a computer to search for patterns that could help with segmentation, and that you can use to predict key customer behaviors.
STEP 1: IDENTIFY A GOOD PROBLEM
PAYGo businesses are data-rich — products, payments and agent touch points generate information that machine learning can use for informing key decisions. As a first step, it’s important to identify areas of the business where an existing or potential prediction or decision is complex enough to warrant ML in the first place. The best ML opportunities are those that have a strong combination of the following characteristics:
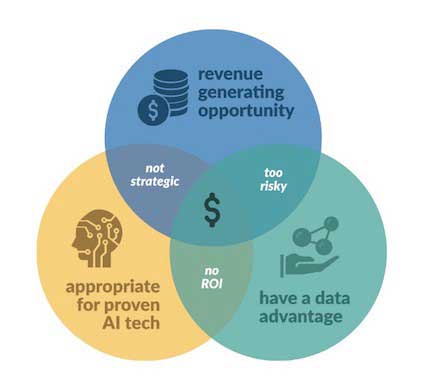
- They are informed by large, relevant, historical datasets: For ML to work, providers need a large historical data set that includes rich features and labels, ideally with high-quality data that is proprietary and is well understood by the company. If you don’t have data, you can’t use machine learning! And if you have a small data set, you might want to pursue statistical techniques instead of ML. At this stage you’re looking at the type of data available (i.e. financial, transactional, product usage and performance, call center, etc.), the resources in place to normalize and store it, and how applicable it is to the current product or business model you’ll be designing for. As we began our engagement, ZOLA’s new three-year lease business model had over 25,000 paying clients and was adding approximately 5,000 a month on the new offering. The company’s databases included historical data on transactions (deposits and ongoing, regular top-ups), product details, customer care interactions, information from sales/installation/service visits, and some product usage data. Together, these data points created a base from which the ML model could be built and trained.
- They use ML to feed into a process/decision that’s important for a priority problem: With the excitement and hype around Artificial Intelligence and ML, it is tempting to jump in looking for a place to use machine learning. This approach is a mistake. First, providers need to find a problem in their business that matters immensely, and could have a high likelihood of being solved. Ask yourself: What mission-critical information are you dying to know? What problems could you solve “if you only knew ___?” Minimizing non-payment and customer churn are critical, ongoing challenges for PAYGo operators that dramatically reduce expected revenue if left unchecked. So for ZOLA, we identified a clear area that could be significantly improved with accurate and timely predictions of repayment behavior: The decisions of who to target for better repayment, at what point in the customer journey to reach out to them, and with what tools.
- They have the potential to grow revenue and/or reduce costs: It’s important to start with an initial assessment of the ways in which the proposed ML model might deliver cost savings. These might include: lowering the cost of prediction and forecasting; managing repayment risks and lowering defaults; expanding serviceable customer segments; identifying and delivering on new up- and cross-selling opportunities; and delivering differentiation — i.e. through automation, customized communications and offerings — along with a better overall customer experience. It’s also important to think about how these financials could evolve in the next two to three years, considering your growth and anticipated changes in the market. At a later stage with a first iteration of the model, you can use these identified revenue and cost opportunities as inputs into a return on investment calculator. Losing customers is expensive for any business. Like most PAYGo operators, ZOLA manages post-sale interactions with customers through call centers and a network of agents, who are focused on post-sales service at the customer’s doorstep. Prior to their work with FIBR, ZOLA’s post-sales interactions were generic and applied uniformly across the portfolio, without regard for the product, repayment behavior, age or churn risk of the individual customer. We saw significant opportunities to both reduce outright churn as well as eliminate interactions with clients who do not need them (because they would likely pay on their own), by better targeting the right people at the right time in their customer journeys.
- They can be tested quickly, using proven, widely-available AI tech: In ZOLA’s case, there is a large, existing literature and experience base on how to increase customer retention. Churn prediction is a common ML use case in financial services, telco and digital technology companies, so ZOLA could take advantage of existing wisdom and lessons learned. For decisions/problems where there is not a relevant base of experience, being the pioneer can be more costly and difficult.
- They are addressing problems for which the solution is not urgent: Specific timelines will vary by provider and use-case, but we have found that it’s safe to budget four to six months to deploy a new ML solution. Any less and the solution will not be properly implemented or tested. If the problem being addressed requires a decision in a shorter time frame, such as a few weeks, ML is not a useful tool. It requires cross-functional team collaboration, multiple model iterations, and often changes to company processes or decision-making — all of which require sufficient time and development to be useful.
To get you started, here are a few examples of machine learning in practice that are relevant to companies deploying a PAYGo model. For each potential ML use case, we have identified the core problem that would be addressed, described what might be predicted and the type of data that might be used for a prediction, and discussed what process or decision-making the predictions could improve:
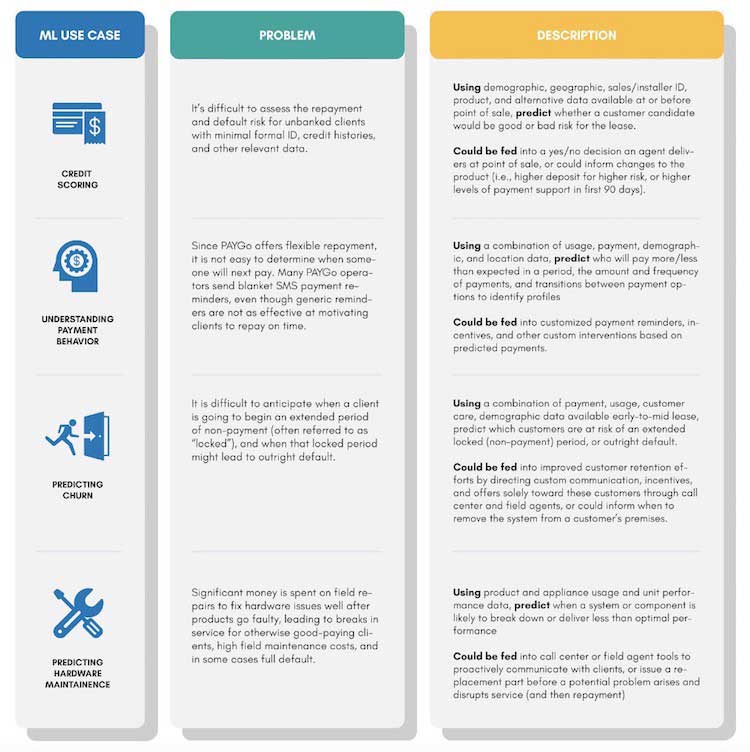
STEP 2: NARROW YOUR FOCUS TO A SINGLE PREDICTION QUESTION
To begin exploring AI/Machine Learning and data without a concise question in mind is akin to acquiring a room full of tools and materials without knowing what you’re going to build. Before jumping into data exploration and modeling, it is crucial to establish a primary question that will guide your efforts. This question needs to be driven by the specifics of your individual business.
In ZOLA’s case, with the PAYGo solar systems it sells on a three-year lease-to-own model, the company allows customers to frequently make payments in amounts of their choosing. But it strongly advocates for monthly installments to improve portfolio predictability, and to minimize friction in making payments using mobile money. While payment flexibility may be attractive to end-consumers, ZOLA and other PAYGo operators need to keep periods of non-usage within reasonable limits to ensure that leases are completed in a timely fashion. To this end, ZOLA deploys escalating interventions after a solar system is “locked” due to lack of payment. The company typically deploys an agent to a customer’s home to follow up on non-payment, and sometimes repossesses the asset after it has been locked for a certain number of consecutive days. So after reviewing the studies ZOLA had conducted internally and considering the business context, we determined that our prediction question should be: “On any given day, predict what inactive customers are at risk of x consecutive days locked.”
This question served as our initial guide for ZOLA’s churn prediction effort, because it’s the timeframe that is most relevant for their business model — it matches their billing preference for clients, it’s a timeframe in which the company can take action, and research and exploratory analysis indicates that once a client is locked for this period, their likelihood of reactivating and catching back up on payments becomes much lower.
STEP 3: HAVE A PLAN FOR IMPLEMENTING PREDICTIONS
Just as it is important to determine what decision you want to consider, you must also decide what you are going to do with your insights. Once you have identified a prediction/decision where ML can add value, and organized yourself around a single prediction question, you will need to think ahead a few steps to build a shared vision of how the prediction will feed into processes, product changes and decision-making. Think about who will receive the prediction the ML process delivers, how they will receive it, and what action they might take as a result. Otherwise you’ll end up with a cool technology that doesn’t address a real user need.
In ZOLA’s case, predictive modeling and machine learning ultimately reduced churn and could deliver a positive ROI only once it was paired with interventions that we designed and field tested to reduce repayment risk. Here are some examples of operational uses of churn predictions from our brainstorm with ZOLA that could be equally relevant for other PAYGo businesses:
- When a call center representative sees the likelihood that an individual customer will churn (as a percentage) in the customer relationship management software, the script of that conversation can be adjusted accordingly. For example, any inbound calls from high churn-risk clients will receive a different script, including questions to understand the reasons why they are locked, and an offer for either a top-up loan or to downgrade to a smaller product.
- Churn predictions are used to create a list for call center representatives to conduct daily outbound person-to-person payment reminders and/or lease renegotiation conversations.
- Field agents receive a list of predicted-to-churn clients each evening, which is used to plan phone calls and customer visits the next day, prioritizing high-risk clients with targeted messaging and offers.
- Finance departments use the predictions to determine whether to remove clients at risk, evaluate if the hardware can be refurbished and redeployed, or make special offers to recuperate high-risk clients.
- Churn predictions are also used to automate the decisions of who receives an intervention, at what point in the customer relationship and in what format (i.e., outbound calls, robo calls, automated and custom SMS interactions) the intervention happens, and the type and timing of visits by a ZOLA agent.
NOW WHAT?
Once you have finished these three steps, what should you do?. We’ll answer that question in an upcoming post, in which we will explore how to: organize and create new features from your data, perform exploratory data analysis, set up in a live test environment, and build and iterate on an ML minimum viable product — that is, a first version of a predictive model whose results can be evaluated against the baseline.
What if I’m not ready for ML yet?
Don’t worry! Most businesses will find that maybe five to 10 percent of their data is correct and useful for an ML push. ML might not be easy to set up, but the potential efficiency gains are so substantial that it is not an approach many businesses can leave off the table for too long. Here are two timely steps you can take now to get yourself ready for ML in the future.
Keep reading about how to use AI in your business:
- Read FIBR’s “Artificial Intelligence: Practical Superpowers — The Case for AI in Financial Services in Africa.”
- Explore the practical applications of AI demos for merchants and PAYGo providers by visiting the FIBR AI Gallery.
- Evaluate your AI Readiness with the AI Readiness Toolkit from the BFA program, Catalyst Fund.
Start to organize yourself to be data-driven and data-ready:
- Log everything, build scalable storage and processing systems, start to conduct deep analysis and link to field experiments with your products and customers.
- Collect more data at point of sale, and ensure product offering and customer journeys are tracked closely — and that data related to these are structured to enable future analysis.
- Establish processes for ensuring the validity, accuracy, completeness and consistency of data. For example, sociodemographic data collected in the field by agents using a smartphone app might be audited by a phone call from a PAYGo operator’s call center rep to the end-customer.
This blog post was originally published on NextBillion
View the PAYGo NEXT Innovation Gallery Demo Presentations
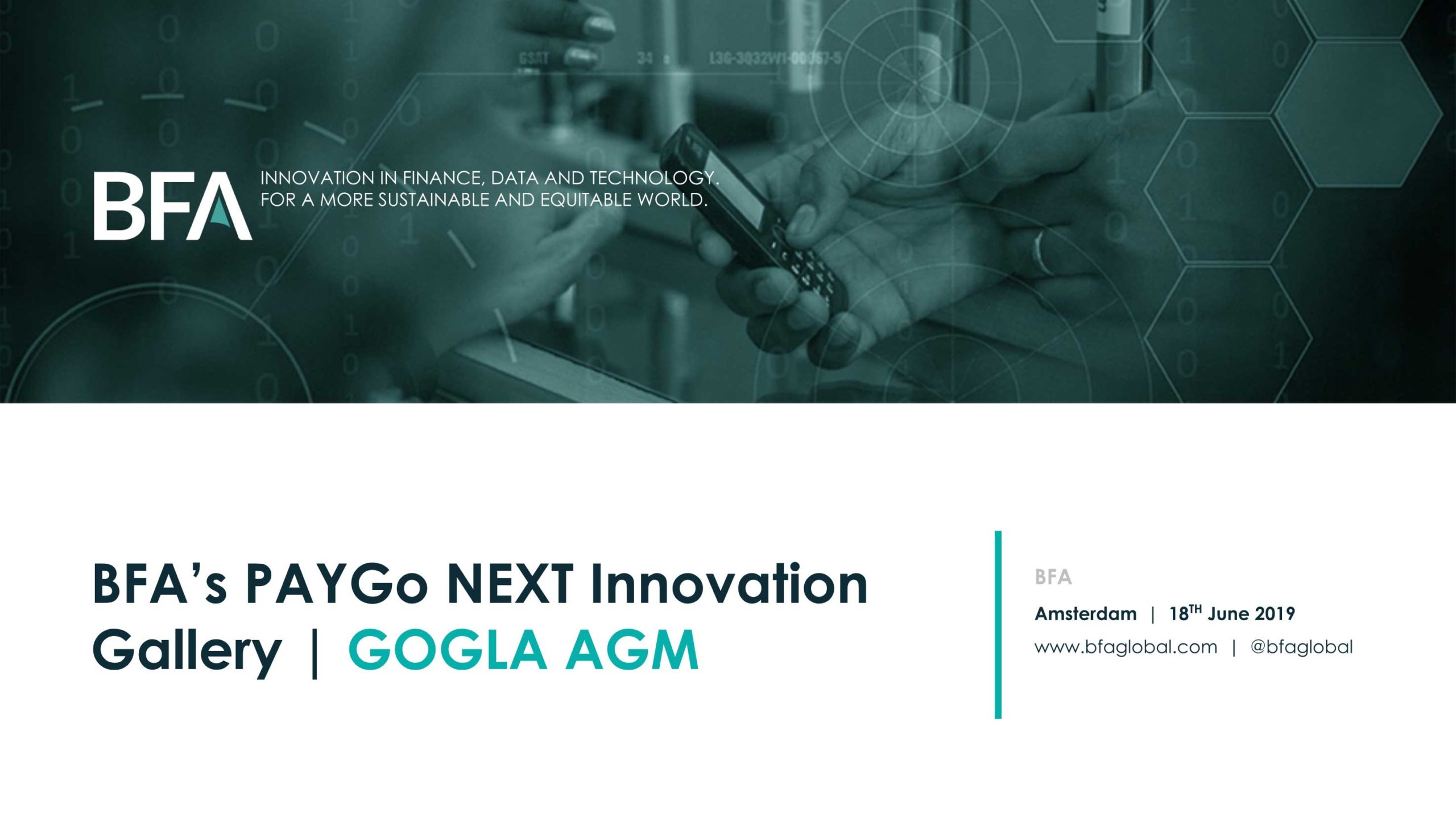
See BFA’s demos for PAYGo operators such as:
- Churn prediction models to help identify customers at risk and better target customers for repayment
- Robotic process automation (RPA) to streamline time-consuming backend processes and examples of chatbot for agents
- Geospatial maps to visualize potential markets for expansion using predictive analytics and public data set
- An app for optimizing field operations for agents to reduce cash transfer risk