The Emergence of ‘SupTech’ — And How it Can Move Past the Experimentation Stage
“SupTech” is a word you’re likely to hear more about in the coming years. The term is a newfangled portmanteau of “supervisory” and “technology” that describes innovation in financial supervision. The application of Big Data techniques and Artificial Intelligence (AI) to the supervision of financial service providers in areas such as consumer protection is radically improving the oversight capacity of central banks and other financial authorities, while lessening the regulatory burden for providers. The potential for this to further financial innovation and inclusion has been widely researched (see a recent report from UNSGSA, for instance).
As the field develops, questions are emerging about exactly what SupTech includes — and what it doesn’t.
For instance, there’s one question that comes up again and again in discussions about SupTech: What distinguishes it from ordinary upgrades by financial authorities to their information communication technology (ICT)? For example, is switching from email to web portals for the submission of regulatory returns an instance of SupTech innovation, when the reporting requirements and format (typically Excel) remain the same? Similarly, can the deployment of a new data warehouse be described as SupTech, if the underlying data previously dispersed among multiple databases doesn’t change?
For many capacity-constrained authorities that we have engaged with at the RegTech for Regulators Accelerator (R2A), a pioneering accelerator program administered by BFA Global, such improvements can indeed unlock substantial efficiency gains. Often these are the necessary precursors of more transformational projects involving Big Data and Artificial Intelligence. But do they qualify as SupTech?
DEFINING THE ‘SUPTECH GENERATIONS’
I attempted to answer these questions in the newly-published report, “The SupTech generations,” co-authored by Jermy Prenio and Stefan Hohl of the Bank for International Settlements (BIS), and my colleague Simone di Castri. Prenio and Dirk Broeders’ landmark paper in 2018 set the groundwork for the study of this still-nascent field. In it, they laid out a taxonomy of SupTech applications, and identified a number of initiatives launched by central banks and other financial authorities worldwide. With this second paper, we surveyed 38 institutions about their SupTech ambitions. Around one-tenth of the responses clearly fell in the “ordinary ICT upgrades” bucket, highlighting the ambiguity that continues to shroud this concept, even among practitioners.
In order to clearly demarcate the SupTech frontier while still giving due consideration to less-innovative — but still transformative — efforts, we propose a framework that categorizes technology for supervisors into four generations:
- The first generation involves data management workflows that are heavily manual and analytics that are mostly descriptive. Think of manually entering data into spreadsheets, spot checking for errors, storing those data in disparate databases or folders, and laboriously transforming data to generate static management reports. This has been the starting point for most financial authorities, and remains the reality for many today;
- The second generation covers the digitization and automation of certain paper-based and manual processes in the transmission, processing, storage and visualization of data. Improved analytics allows for more thorough diagnoses of changes in the supervisory landscape, as well as richer descriptive insights than were possible under the first generation;
- The third generation covers Big Data architectures. These architectures are built with technology stacks that support data of higher granularity, diversity and frequency than could be accommodated previously. Greater computing power coupled with larger data pools enable more advanced statistical modeling, including predictive analytics;
- The fourth-generation involves the addition of AI as the defining characteristic. Generally, AI-enabled solutions or tools presuppose an underlying Big Data architecture, since most AI models require large volumes of data and significant computing power for their results to be valid, meaningful and actionable.
SupTech straddles the third and fourth generations. Figure 1 below lays out the technology stacks of each generation:
Figure 1. Generations of SupTech

This conceptualization can be useful for understanding the patterns and pace of change in this rapidly-evolving field. The authorities on the cutting edge of SupTech tend to have passed through earlier generations before pursuing more ambitious Big Data-driven and AI-enabled data architectures. For example, the UK’s Financial Conduct Authority is seeking to implement digital regulatory reporting at a time when its Gabriel online reporting system is due to retire after nearly two decades of service. Meanwhile, other authorities are only now instituting their own web portals. This suggests that authorities are prioritizing getting their data warehouses in order before graduating to third- and fourth-generation SupTech. To be adequately prepared for this stage, they need a robust data infrastructure capable of handling data flows of increasing volumes, velocities and varieties. This puts an even greater premium on reliable (i.e., machine-driven) validation checks of the data as they are reported.
But even where pioneering supervisors are inclined to leapfrog to the SupTech frontier, they will find themselves in mostly-uncharted territory. The survey reveals that SupTech is still in its infancy, with around one-third of reported initiatives in experimentation, and another third under development. The overwhelming majority of the projects were launched in 2018 and 2019 — this is still the early days.
EARLY FOCUSES OF SUPTECH: REPORTING AND MISCONDUCT
As illustrated in Figure 2 below, reported initiatives to date cluster mostly around reporting and misconduct analysis use cases, arguably the low-hanging fruit of SupTech. Automated reporting pertains mostly to the use of application programming interfaces (APIs) for machine-to-machine transmission of regulatory returns. They form the foundation of many Big Data architectures and the backbone of advanced analytics applications (often based on machine learning) that rely on plentiful data to generate meaningful results. For many, this is the logical starting point on the SupTech journey.
Figure 2. Distribution of Surveyed Suptech Initiatives by Use Case
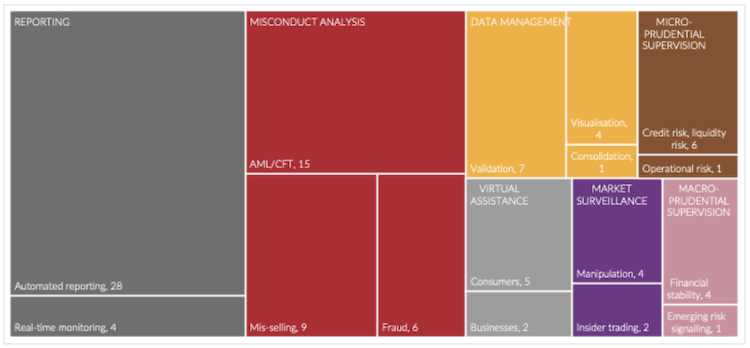
Misconduct analysis leverages a mix of anomaly detection, text mining and other machine learning techniques to catch and combat money laundering, terrorist financing, miss-selling and fraud. These use cases lend themselves well to data mining thanks to the ready availability of massive, granular, labeled transactional datasets kept by banks and other payment system operators. Add to that the growing demand from authorities struggling to keep up with rapidly-evolving digital financial services, plus the pressing need to counter de-risking in the financial sector, and the prevalence of misconduct use cases makes perfect sense.
IS SUPTECH STUCK IN EXPERIMENTATION MODE?
The slow pace of SupTech adoption, at least compared to the breakneck speed of change in the fintech space, is partly a reflection of the strategies devised to develop SupTech initiatives. Only about one-quarter of respondents reported that they have established a SupTech roadmap (ie: a plan that charts out the design, development and deployment of solutions in carefully-sequenced phases). Most others either do not have an explicit strategy, or subsume SupTech under “digital or data transformation programs,” which focus more on second generation-type improvements to supervisory data architectures.
Still others use innovation hubs or labs where in-house teams tinker with SupTech applications in a trial-and-error fashion. Nearly half of all initiatives rely on internal resources to develop their solutions, rather than external partners or vendors. This approach has the advantage of tapping into institutional knowledge and expertise, while creating a safe space for experimentation. But it might also miss key non-technical ingredients, such as the market intelligence and competitive pressure that make fintech startups so fast and disruptive. That is not to imply that innovation does not occur in these hubs — the survey clearly shows that it does — but rather that it may help account for the halting advance of SupTech innovation.
One promising middle way is R2A. It enlists the help of outside technologists and SupTech experts to co-create solutions along the lines of a traditional accelerator. The aim is to de-risk engagements between financial authorities and innovators and create proofs-of-concept, prototypes and full-scale solutions in rapid time and at minimal cost. To learn more about how this is achieved, see the R2A process or visit our website at www.r2accelerator.org/.
Originally posted on NextBillion