How does inclusiveness translate to usage of transaction products? Comparing apples to oranges
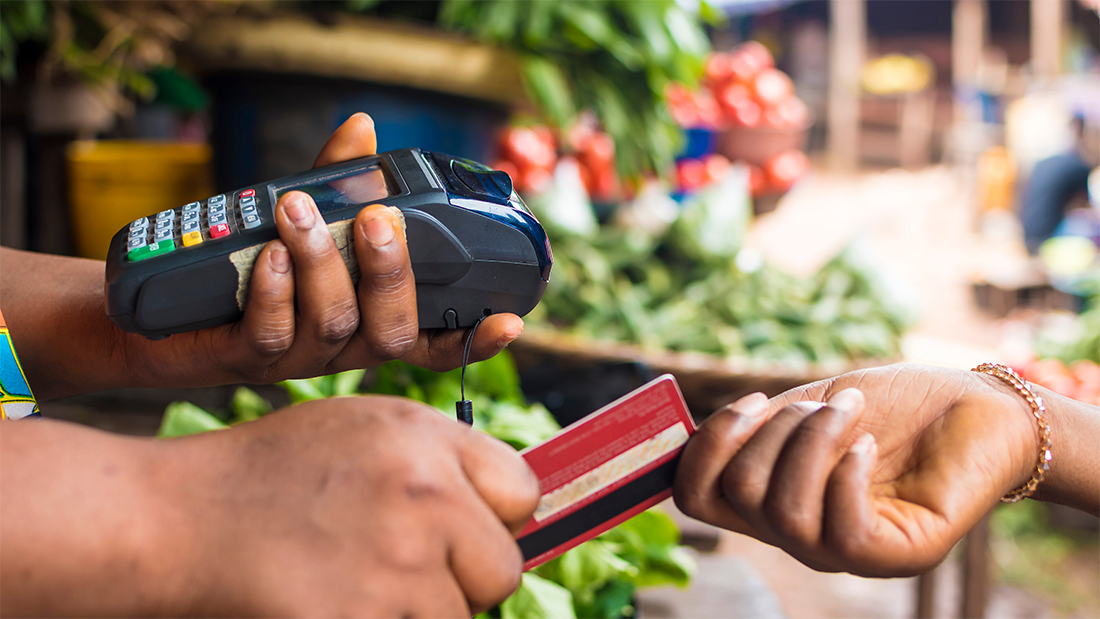
This is the fourth case study in our report, ‘Inclusion and Your Bottom Line’, which outlines a new mixed-method approach to characterizing and measuring inclusiveness for financial products and services.
In the past, financial behavior – or customer usage of transaction accounts – has been based on relatively simplistic metrics such as: the frequency with which one makes withdrawals or deposits, the amount of those transactions, the length of time that one holds a balance (or approximate balance) in their account, etc. But savers, dormant account users, and those that only use their account for withdrawing paychecks come in all shapes and sizes. One can both withdraw and deposit irregularly and in small quantities, yet still be saving towards a goal. Conversely, just because a customer occasionally deposits large sums of money does not necessarily mean that they are accretively saving towards some sort of goal.
One of the aims of a recent project – along with many that BFA Global engages in – was to quantitatively define some notion of intent when it comes to a customer’s financial behavior over time. Are they typically saving towards a goal, or maintaining a relatively steady balance in case of emergencies, or rapidly withdrawing their funds as soon as they receive a paycheck?
Consider a simple example. Suppose Akinyi saves exactly 2,000 KES every other week for six months, while Bishara saves somewhere between 1,000 KES and 10,000 KES at some point during the month for a year. We would consider these behaviors similar because they indicate the intent to accumulate funds in order to reach a goal of some kind; yet the specifics of the balance growth differ. See the plots below, which illustrate these two behaviors.
While they differ in the specifics, one can see how the shapes of these plots are broadly the same and can be characterized as such.
Now consider two different savers with seemingly very different behavior. Carmen saves irregularly, but quite often and with large sums of money. Furthermore, she withdraws small amounts of money every once in a while from her account. Declan – on the other hand – only saves very small amounts, relatively infrequently.
While both individuals exhibit characteristics of someone who is accretively saving funds, there is not a straightforward rules-based method for determining that. For example, while Carmen is clearly saving money, she withdraws every once in a while. Similarly, Declan’s balance barely increases over the course of the year, yet he is indeed saving.
BFA has historically used unsupervised learning – a type of machine learning – to group together similar types of financial behavior, such as these. The general observation is that there are about 5-6 savings behaviors that all customers can display at various points in their financial lives. Broadly speaking, they can be categorized as: savers, balance sustainers, fast drawdowns (those who quickly deplete savings), slow drawdowns (those who gradually deplete savings), and dump-and-pulls (referring to those who simply use the account for withdrawing their paycheck). These are behavior types that are not easily defined by amounts of frequency of deposits/ withdrawals alone. In order to characterize these types of financial behavior amongst thousands or even millions of clients, we needed to form a further basis of comparison across different periods of times holding a certain balance, frequency of deposits/withdrawals and quantity of funds.
The first step the team at BFA Global took was to normalize the customer balance histories across a financial institution such that they were time27, deposit/withdrawal frequency, and quantity agnostic. That is, we ran calculations on all of the balances at the institution to “smooth out” small differences between balance histories. We then clustered those normalized balance histories together into the five different clusters mentioned earlier to see which ones had similar, high-level characteristics. Through this process, we are able to deduce which customers have similar financial behaviors, regardless of how often they get paid or how much their paycheck may be worth and the age of their accounts.
This is important on many levels. First and foremost, both financial institutions and donors are interested in affecting customer behavior, and it’s quite difficult to do that without knowing what that behavior is. Secondly – for the institutions in particular – the user behavior can be directly connected to the business model to determine which types of behavior are contributing to or detracting from profitability and efficiency.
Finally, it helps to identify which low-income customers are saving for a goal or maintaining a balance for emergencies, even though they may not be able to save as much. This is an important data point, for instance, for thin-file customers who require evidence to show that they are creditworthy.
READ MORE
Inclusion and your bottom line
A new mixed-method approach to characterizing and measuring inclusiveness for financial products and services, as carried out in Kenya, South Africa and Nigeria.