Keeping Machine Learning Honest! The need for the truthalizer
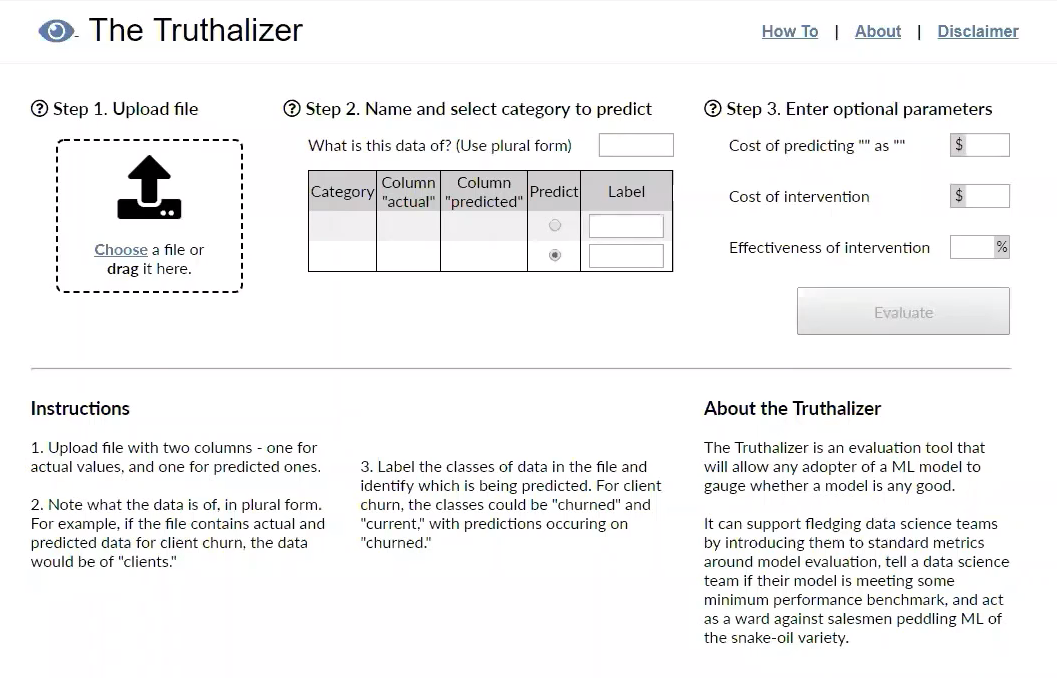
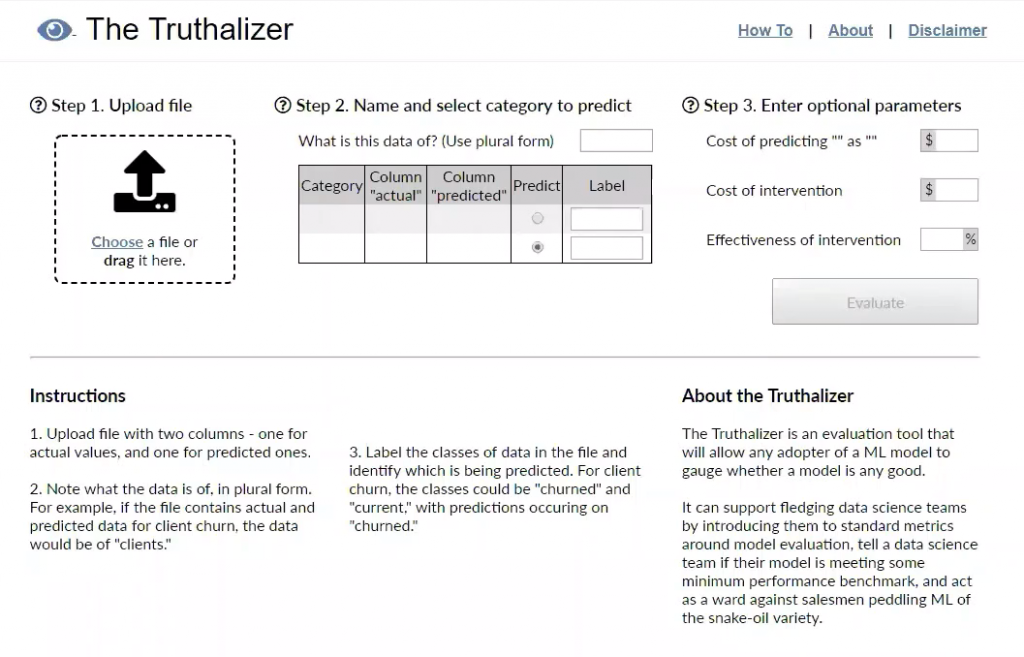
Machine learning (ML) provides institutions with predictive analytics that leverage large amounts of both historical and continually-generated data to obtain actionable insights. These insights, in turn, allow institutions to design and deploy interventions to increase revenue, lower costs, and compete in a dynamic marketplace by providing more customer-centric products and services.
However, few understand or have visibility into the inner workings of machine learning models so many are left wondering how good the insights actually are. For skeptics and experts alike, BFA has created the Truthalizer, a verification process that uses an out-of-sample dataset to evaluate the efficacy of a particular machine learning model as well as evaluate the intervention it triggers. As such, the Truthalizer can help leaders understand the quality of insights and how much to rely on them.
For example, the Truthalizer could help in any of the following scenarios:
- Alice is a burgeoning data scientist. She has worked through the introductory exercises offered by Kaggle, but much remains a mystery. “Which one is precision, and which one is recall,” she wonders out aloud, “and why is the Confusion Matrix itself so confusing?”
- As head of the Data Science team at his company, Bob is promoting a new model to predict customer churn. Compared to the current model, the new model has different tradeoffs between false positives and false negatives. “We have three interventions to prevent customer churn, and their costs are different, as well as how often they actually work,” his department head tells him. “Will your new model actually save us more money?”
- Carol believes that ABC Predictive Analytics Company could be game-changing for the healthcare division she oversees. Her gut, however, tells her the promised results sound too good to be true. Her Quantitative Analytics team does not have access to the proprietary algorithms that drive ABC’s predictive engine. “Are they for real, or are we being taken for a ride by the snake oil salesman of the digital age?” wonders Carol.
Each of these are variations of the question: is the machine learning model any good? At BFA, we hear this question often from our FIBR partners that use ML techniques. The question is a good one because, in our experience, the process for verifying the model is often a weak link in setting up ML, whether in-house or in partnership with an external service provider.
The Truthalizer answers this question by using an out-of-sample dataset and cost data to: 1) assess the efficacy of a particular model using standard evaluation metrics, and 2) generate return-on-investment calculations to evaluate the intervention triggered by the model.
The Truthalizer is housed at truthalizer.ai. Note that the Truthalizer does not actually run any models or undertake any computations to derive predictions; these are left to the user.
How Truthalizer can create business impact
Machine learning models can be evaluated on the frequency and costs of incorrect predictions, namely false positives and false negatives. The Truthalizer allows firms to evaluate the costs and benefits of machine learning by allowing different costs to be assigned for false positives and false negatives to accurately assess the impact of the model to the bottom line. With a better understanding of the impact on the budget, leaders can make better decisions about whether/how to deploy machine learning (ML).
Furthermore, the Truthalizer can help fledgling data science teams by introducing them to the best practices of model evaluation, and by providing a disinterested evaluation to executives and champions on the merits of a model. By combining both evaluation and return-on-investment (ROI) calculations on the same page, we hope it encourages data scientists to keep ROI considerations in mind and enables non-technical executives to understand more of the underlying technology.
We also expect the Truthalizer to act as a ward against salesmen peddling ML of the snake-oil variety. Sub-par ML implementations will provide predictions that are worse than those derived from more basic techniques, ultimately costing more than if they were not used. The tool will help users understand that sometimes bad ML is worse than none.
As such, we hope the Truthalizer will remove much of the risk associated with incorporating predictive ML models for FIBR partners and other financial service providers (FSPs).
Truthalizer in Action
The Truthalizer is designed to calculate ML evaluation metrics, as well as display and explain them. These evaluation metrics consider the rate at which the model generates false positives and false negatives. For example, many providers consider using ML to predict whether a loan will remain current or become delinquent; in this case a false positive would be a loan that will remain current but the model predicts will go delinquent and a false negative is a loan that will go delinquent but is not predicted to be so.
Consider an example:
There are 147,150 loans in the uploaded file. Of them, 4,724 are late and 142,426 are current. Continuous predictions detected. Cost of predicting incorrectly is $100, cost of preventative intervention is $10, and the effectiveness of that intervention is 60%.
1) First, the Truthalizer presents a confusion matrix, a contingency table that compares predicted results with actual ones. This table forms the basis for evaluating the model.
The confusion matrix and evaluation metrics change their results dynamically based on the threshold chosen. A 50% threshold value is the default to differentiate between current and late loans. Since the cost of a false negative is not equal to the cost of a false positive, the Truthalizer offers two thresholds for evaluation — one that maximises accuracy, and one that minimizes cost. More on this below.
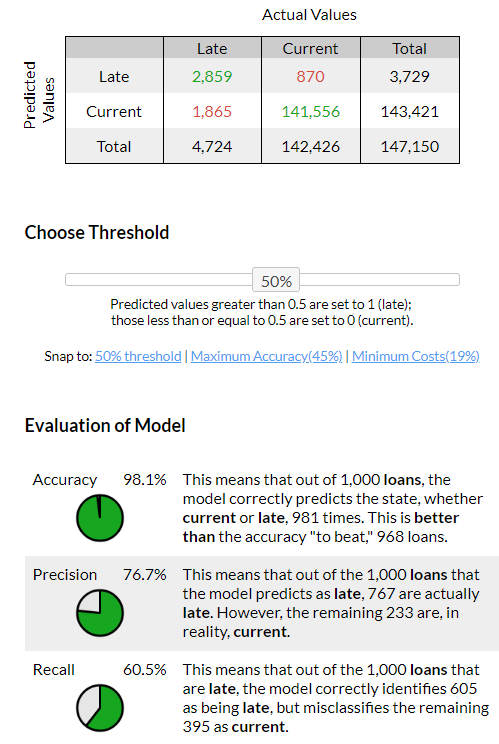
In an ideal world, the model would predict current loans as current, and late loans would be predicted as late. In the real world, we hope to minimize the number of false positives and false negatives, which are indicated above in red.
The table that accompanies the confusion matrix displays and explains three evaluation metrics: accuracy, precision and recall.
In the example above, the Truthalizer explains a recall result of 60.5%: “This means that out of the 1,000 loans that are late, the model correctly identifies 605 as being late, but misclassifies the remaining 395 as current.”
2) With this evaluation of the ML model completed, the Truthalizer uses data about the unit costs of servicing loans (entered independently) to consider the cost efficiency of an intervention to prevent default. It generates a detailed worksheet that walks users through the return-on-investment for such an intervention. The calculations reflect the fact that the cost of a false negative is not equal to the cost of a false positive.
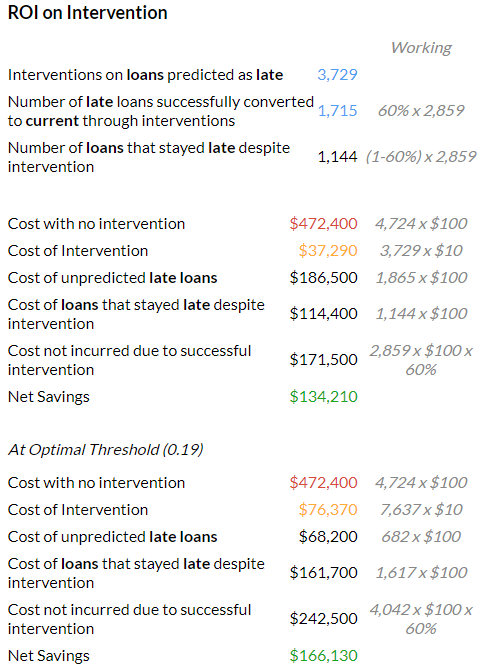
In the example above, we assume that the cost associated with a late loan is $100. This cost could include delayed interest income, loss of principal, as well as expenses associated with chasing down repayment. It also assumes that interventions exist that can prevent a loan from going late, but that they cost $10 per loan, and are effective 60% of the time.
With these data inputted, the Truthalizer calculates savings created by the intervention as follows:
Savings = Cost with no intervention — (Cost of unpredicted late loans + Cost of loans that stayed late despite intervention + Cost of intervention)
In this case, it shows a savings of $274,450.
For continuous prediction values, the tool calculates and presents two threshold values, one that maximizes accuracy and one that maximizes savings, allowing users to snap to those values (Figure 1).
Finally, the ROI evaluation includes two graphs that display how the various costs and savings change if the threshold levels for the model are changed. They allow users to explore how the impact to the bottom line changes if the false positives and false negatives have different costs associated with them.
The FIBR Impact
BFA created the Truthalizer because we could not find a user-friendly tool for our FIBR partners that allowed for approach- and model-agnostic evaluations, and explained model performance in relatively simple terms. The Truthalizer delivers on our learning agenda by allowing FIBR partners to:
- Adjudicate onboarding ML models to ensure that ML-based prediction models perform better than some other approach, such as expert systems
- Compare ML models to provide a consistent baseline against which to compare competing ML approaches and corresponding models, especially over time
- Evaluate Partnerships to independently evaluate claims made by a vendor on the efficacy of their offering
Our goal is to boost confidence in predictive models that use existing and new data. By providing a reliable evaluation of the power of these models, we hope the Truthalizer encourages businesses to leverage the data they currently generate and perhaps digitize further to generate more data.
Furthermore, the Truthalizer can help convince financial service providers (FSPs) to work with FIBR partners to “offer, fund or underwrite financial services to low-income end-users through the local businesses they are associated with”. First, it will quantify uncertainty for FSPs by reliably predicting default, churn, or any other future event of interest so FSPs can price risk of engagement with FIBR partners appropriately. Second, FSPs will be better able to predict future behavior so as to offer appropriate products and services to customers, thereby allowing them to segment customers for upsell or cross-sell.
Further, Catalyst Fund’s AI Readiness Toolkit can help startups evaluate and prepare for implementing AI more broadly.